Data lakes vs. data warehouses
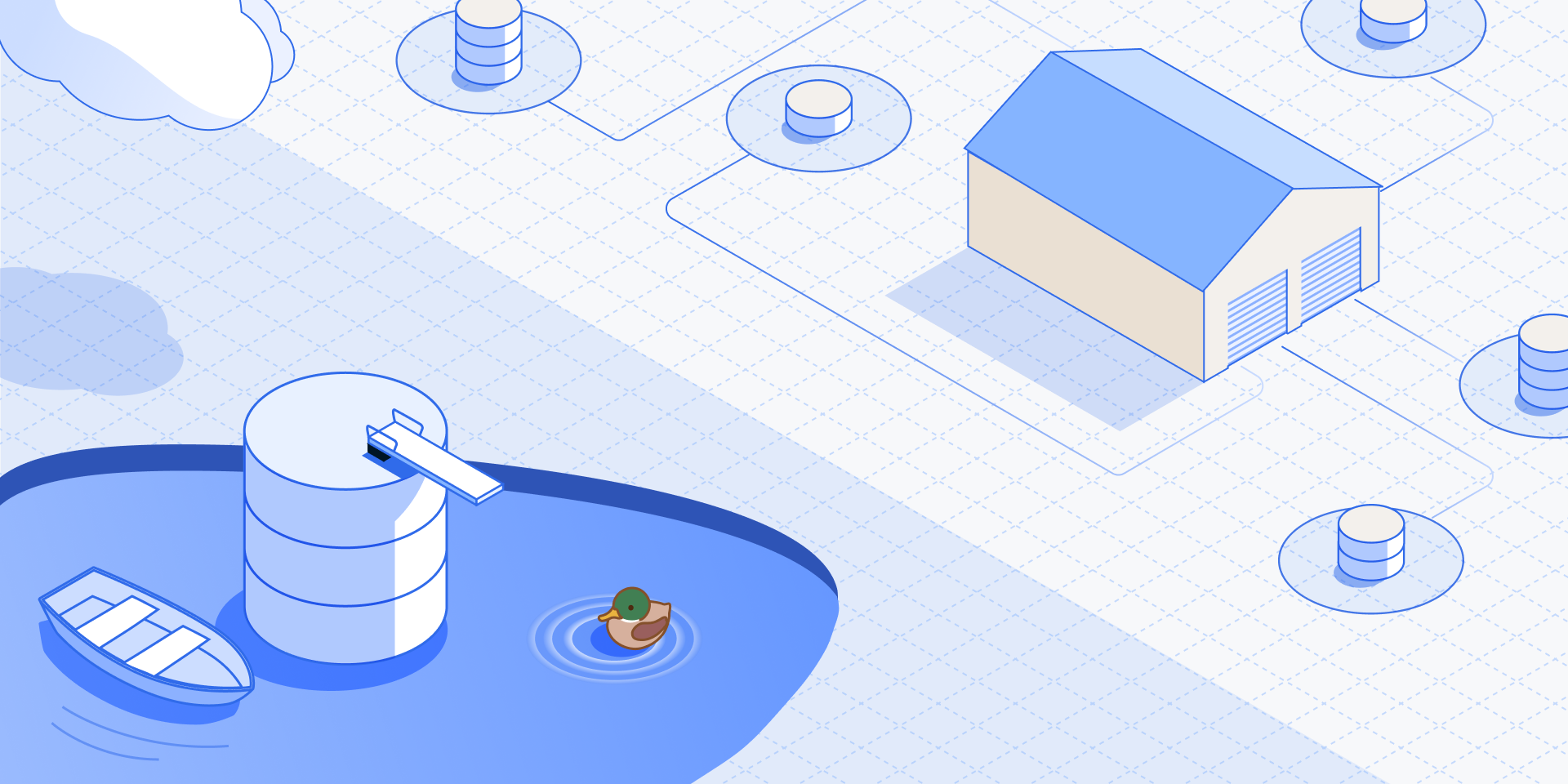
In a survey conducted by IT consulting firm Capgemini, 77 percent of enterprises said that decision-making in their organizations was completely data-driven. The same survey showed that only 39 percent of respondents were able to turn their data-driven insights into a sustained competitive advantage. Data-driven decision-making only works when you have access to the right data at the right time, which 69% of respondents find challenging.
Organizations use data lakes and warehouses to store large amounts of data, which they then access through business intelligence and data science tools to gain insights and make decisions. When used correctly, your data warehouse or data lake can support you with timely, accurate decision-making. Let’s take a detailed look at both technologies.
What is a data warehouse?
A data warehouse is a large, central repository of structured data. It receives business data from a variety of sources in a structured, predefined format and readies it for reporting and analysis.
How does a data warehouse work?
Data warehouses are generally associated with extract, transform and load (ETL) data integration, in which data is extracted from the source, normalized or transformed into a standardized format and loaded into the warehouse. It usually receives data from:
- Databases, including both relational database management systems (RDBMSs) and semi-structured NoSQL databases
- Enterprise resource planning (ERP), customer relationship management (CRM), and other Software-as-a-Service (SaaS) applications
- Event streams
- Files
Data warehouses use a schema-on-write model where incoming data is checked for compliance with the existing schema. Data stored in data warehouses is curated, centralized and ready for use.
Why use a data warehouse?
Since data warehouses store data that’s ready for analysis, both technical and non-technical users can benefit from it. Data from a data warehouse is mainly used in business intelligence, data visualization and batch reporting. Data analysis tools can directly connect to a data warehouse, and data teams often automatically schedule reports and dashboards, such as monthly sales reports. and business users can access them as needed.
How is a data warehouse different from transactional or operational databases?
Data warehouses differ in design philosophy from transactional or operational databases, which perform frequent queries and updates to individual records. An example is adding, removing and purchasing items from a cart on an ecommerce website. By contrast, data warehouses are designed to perform calculations across large groups of records at once. This basic difference in design means you must not use the two interchangeably, as they are optimized at a basic structural level for fundamentally opposite kinds of operations.
In the past, data warehouses were hosted in on-premise data centers. Data warehouses are now increasingly based in the cloud. The most advanced cloud-based data warehouses are “serverless,” meaning that compute and storage resources can be independently scaled up and down as needed. Modern cloud data warehouses have become extremely accessible to organizations with modest resources.
Modern cloud data warehouses easily integrate with business intelligence platforms through which analysts access business data to produce reports and dashboards. Also, data warehouses offer a fine degree of user permissions and access control, an essential feature of data governance.
What is a data lake?
A data lake is a large repository of structured and unstructured data. It can store data from any source and in any format so that the data can be used for data discovery, advanced business intelligence and machine learning.
How does a data lake work?
Data lakes are typically paired with the extract/load/transform (ELT) method of data integration — i.e., the data is extracted from the source(s), loaded into the lake and transformed as needed. It uses a schema-on-read approach where the data is given structure only when it is pulled for analysis. Unlike data warehouses, which only accept structured data and enforce schemas, a data lake can accept data from all kinds of sources.
Data lakes store all types of documents and raw data such as user data, research data, video and media files, application data, medical imaging data and so on — the sky’s the limit.
Traditionally, the data stored in a data lake was not analytics-ready, lacking easy support for SQL-based queries or integration with business intelligence platforms. Another challenge of using data lakes was their potential for “murkiness” arising from a lack of structure and governance. An exceptionally disorganized and poorly governed data lake can quickly become a data swamp, making important data unsearchable and inaccessible.
Modern approaches to the data lake, sometimes called the data lakehouse or governed data lake, include support for open table formats as well as data catalogs. These innovations enable ACID (atomicity, consistency, isolation, durability) transactions and schema enforcement as features to make data less “murky,” better governed and more usable.
Why use data lakes
Data scientists and data analysts traditionally used data lakes for streaming and big data analytics, especially machine learning, where the ability of data lakes to accommodate huge volumes of raw data could shine.
With the development of the data lakehouse or governed data lake, data lakes are now appropriate for more conventional reporting and analytics use cases as well.
The governed data lake offers a path forward
Both data warehouses and data lakes will continue to form the lynchpin of the modern data stack, a suite of tools and technologies used to make data from disparate sources available on a single platform. These activities are collectively known as data integration and are a prerequisite for analytics.
Although the various data warehouses remain viable options as single sources of truth for analytics-ready data, the governed data lake is an excellent choice if:
- An organization’s data needs are anticipated to continue growing in scale, volume and complexity, making the cost advantages and flexibility offered by using a data lake for storage more meaningful.
- An organization wants to simplify its data architecture and eliminate redundancies for cost control and better governance.
- An organization wants to pursue Innovative data use cases, such as those involving generative AI, that require all of an organization’s data in one place.
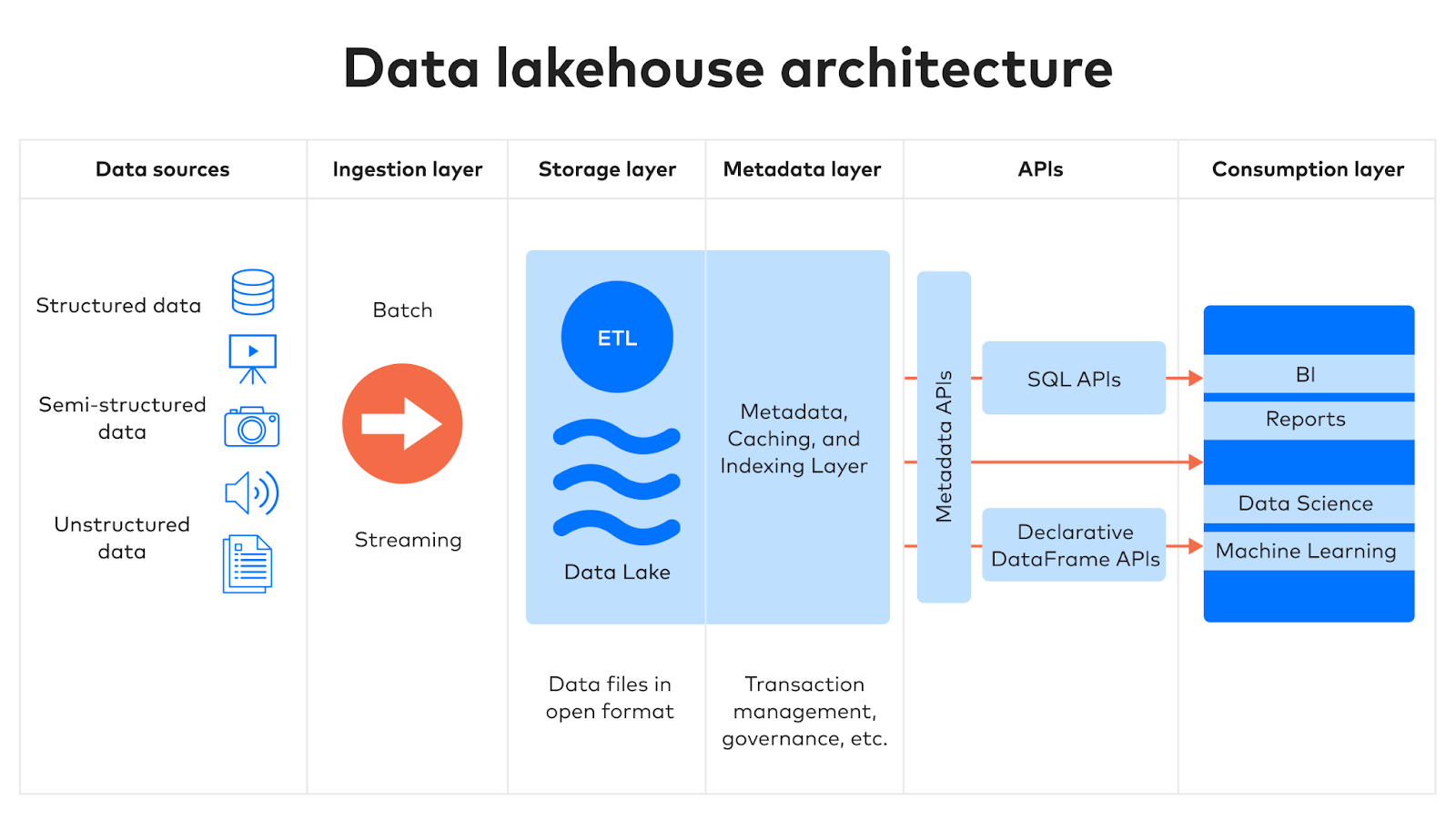
Use data connectors to populate destinations
Data warehouses and lakes are both capable of storing large volumes of data from a wide range of sources. The challenge is data integration – moving data from applications, event streams, databases, files and more to destinations. Despite the seeming simplicity of moving data from one location to another, ensuring that the data is faithfully represented and flows in a timely manner is an architecturally complicated problem.
This problem only grows in scale and complexity as an organization adds more data sources. Building and maintaining a bespoke, in-house solution for data integration is incredibly costly in terms of time, labor and talent.
The answer is to leverage the capabilities of the modern cloud by automating and outsourcing data integration to a provider that specializes in the real-time movement of high volumes of data. Try Fivetran for yourself and experience for yourself how simple and painless data integration can be.
Start for free
Join the thousands of companies using Fivetran to centralize and transform their data.